The Impact of Generative AI on Product Development
As companies experiment with the use cases of generative AI, one of the most promising areas is integrating image generation and text generation tools into the product development process. In response, corporate innovation teams are increasingly focusing on leveraging generative AI to enhance idea generation and creativity, gain market and customer insights, and add user-friendly interfaces to complex systems. In the fall of 2024, researchers Tucker J. Marion, Mahdi Srour, and Frank Piller from MIT Sloan Management Review discussed these possibilities. Their research is based on extensive fieldwork, including dozens of practical interviews with managers. During the study, the team discovered that generative AI can act as a catalyst for transforming and enhancing existing innovation workflows. The research outlines three ways to boost the productivity of innovation teams by integrating generative AI technologies.

Use Case 1: Enhancing Creativity and Design Workflows
Loft, a Boston-based design agency, is one of the many “innovative workplaces” utilizing generative AI in the creative process. In a project that began in May 2023, Loft used GPT-4 to propose new product features by entering existing customer preferences. Additional input was used to identify and refine the most promising ideas. Meanwhile, designers began sketching product concepts, uploading these sketches to the image generation tool Midjourney, and fine-tuning the visual design through prompts alongside their paper work. In these creative stages of the innovation process, the possibility of generative AI generating “hallucinations” that deviate from facts or logic was not a concern, as the team was simply exploring ideas. Such cases are supported by research that suggests generative AI aids brainstorming, leading to more useful human-generated ideas. Once the product development process moved into the design and engineering stages, the tools needed to provide reliable outputs. While publicly available generative AI platforms helped Loft’s team conceptualize ideas and sketch initial prototypes, they paused the use of generative AI tools during the prototype development phase, based on the engineers' chosen concepts. Loft gathered consumer feedback on prototypes through video focus groups and surveys, using generative AI to generate scripts for interactions between consumers and prototypes, which was an area where large language models (LLMs) like ChatGPT excel.
LLMs are capable of summarizing and clustering data, recommending areas for improvement, and identifying features consumers prefer, providing valuable insights for product launch marketing. By integrating these findings, Loft’s design team reflected both general and specific insights about the product into the early design concepts. At this stage, Loft avoided asking the LLM questions beyond the scope of the input data, preventing hallucinations from influencing the analysis. After the first project, integrating generative AI into product development significantly improved Loft’s design process. For example, Loft’s designers quickly generated 50 new toy concepts with various product characteristics using generative AI. Without it, reading feedback from test participants and conceiving new concepts would have taken considerably longer. Generative AI not only accelerated the pace of work but also helped more effectively conceptualize product changes that best met specific consumer needs. The company estimates that generative AI helped reduce product development time by half.
Use Case 2: Using Generative AI for Customer Insights and Concept Validation
Creative Dock, a company based in the Czech Republic, helps create new business units, products, and services by integrating market feedback into various business model concepts before launch. By leveraging large-scale market research data reflecting customer needs in specific areas, the company programmed AI agents to simulate qualitative interviews with potential customers representing specific personas. Some interviews focused on customer needs and preferences, while others sought feedback on alternative value propositions for new product offerings. Using this proprietary data, the tool fine-tuned pre-trained large language models (LLMs) like GPT for specific purposes.
This customized language model allows Creative Dock’s team to use generative AI for idea generation, identifying market demands, and rapid concept testing.
The tool also accelerates the design, testing, and creation of minimum viable products (MVPs). Founder Martin Pejsa reported a 30% increase in development efficiency, a 40% boost in graphic design productivity, and a threefold increase in content creation speed. Generative AI was also used in reviewing all new business model concepts, helping Creative Dock achieve a 50% growth compared to the previous year without hiring additional full-time staff.
FlecheTech, a company based in Switzerland, also uses generative AI to gather customer requirements, but its approach differs significantly. This startup has developed a specialized application for designing and rapidly prototyping printed circuit boards (PCBs), primarily catering to hobbyists, startups, and users without deep knowledge of electrical engineering or PCB design. FlecheTech fine-tuned a pre-trained LLM using a database containing multiple PCB designs and explanations, allowing users to design circuits using simple language like “I want to measure this physical value,” “I want to rotate X at Y speed,” or “I want to communicate with this protocol.”
For complex PCB design queries, the LLM automatically recognizes them and breaks them down into simpler tasks.
Furthermore, customers requiring intricate designs can have their generative AI-supported PCB designs reviewed by FlecheTech’s team before prototyping. The company reports that the time typically required to complete PCB designs—6 to 8 weeks—is now reduced by over 80%. The productivity boost for beginners is particularly notable, expanding the company’s product market. FlecheTech also outsources customer interactions for customized PCBs through a generative AI-based chatbot, achieving significant cost savings.

Use Case 3: LLMs as a Natural Language Interface for Complex Design Tools
The linguistic fluency and ease of interaction with LLMs offer another potential use case as a front-end interface for advanced simulations and engineering systems in product design processes. Siemens’ industrial division recently added generative AI capabilities to its advanced engineering and design software, making it easier for a broader user base to interact with these systems. One such system, Simcenter, is a simulation package capable of accurately modeling the physical behavior of products or processes, replacing physical prototypes and test environments with digital alternatives.
Despite its powerful capabilities, Simcenter typically requires significant setup time, extensive user training, and specialized knowledge to interpret results. Siemens combined a generative AI-based user interface with this tool to develop HiSimcenter.
HiSimcenter can handle a range of tasks, such as answering simple questions to select the most suitable computer-aided engineering tools for a specific job or automatically generating designs based on product requirements. This ChatGPT-based application helped improve modeling efficiency by over 50% by assisting in setting up and running complex simulation models.
However, developing such a system is not a simple task. The engineers who developed HiSimcenter at Siemens recognized the importance of reliable base data and considered securing it as a key challenge when building hybrid expert systems. To develop additional specialized applications using generative AI, Siemens anticipated the importance of training employees to assess the quality of task-related data for key engineering operations. Siemens selected a small team of experts to develop HiSimcenter, ensuring that the output quality of generative AI aligned with Siemens' policies and engineering standards.
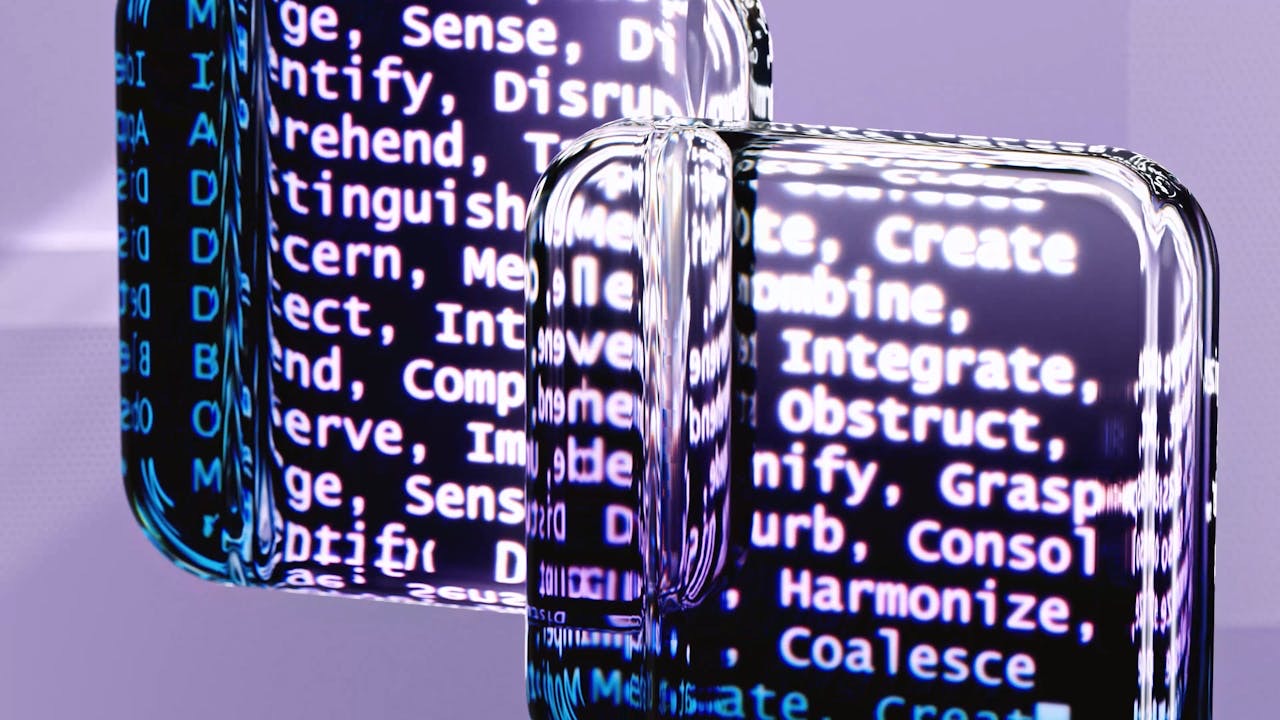
What Does All This Suggest?
Beyond the hype, generative AI can bring tangible benefits to a company’s innovation and development processes.
Managers must consider how the three previously mentioned use cases of generative AI align with their industry and innovation strategy. Moreover, organizations need to have a clear understanding of expectations and desired outcomes, recognizing that different innovation tasks require different approaches. Generally available generative AI tools like ChatGPT or Midjourney, as seen with design firms, are highly suitable for creativity and idea generation.
For more focused applications, such as validating concepts with synthetic personas, pre-trained models should be strengthened with learning data specific to the context. The quantity, diversity, and quality of learning data define trust and significantly impact the quality of generative AI outputs for particular contexts or market segments. When high accuracy and reliability are essential, existing simulation platforms and expert systems are needed. As demonstrated by Siemens and FlecheTech, LLMs can act as efficient user interfaces for such systems, enabling more users with domain expertise to apply these tools to complex engineering or scientific research tasks. When users can handle expert systems directly without the assistance of simulation specialists, they are more likely to run multiple simulations as a tool for discovery, not just validation.
Finally, as these technologies rapidly evolve, the integration challenge within organizations is becoming increasingly important. As Siemens showed, a bottom-up approach with strategic investments in internal development and partnerships is one way to achieve integration. However, these initiatives take time, and due to implementation delays, technologies may already be outdated when they become available.
Therefore, the researchers recommend a democratic, top-down approach where teams and individuals can choose, use, and build the right tools. Their research suggests that combining both approaches allows organizations to strategically build better data and reliable solutions while enabling dynamic experimentation.
'잡학만담' 카테고리의 다른 글
[AI] Career Coaching for the AI Era_ A Special Guide from a Top Mentor (0) | 2025.02.24 |
---|---|
[AI] Empowering the Next Generation_Shaping Tomorrow's Talent in the Age of AI (0) | 2025.02.24 |
[명화] 최후의 만찬(레오나르도 다빈치) (0) | 2025.02.16 |
[명화] 베아트리체 첸치의 초상(엘리자베타 시라니) (0) | 2025.02.14 |
[명화] 밤의 카페 테라스(빈센트 반 고흐) (0) | 2025.02.14 |